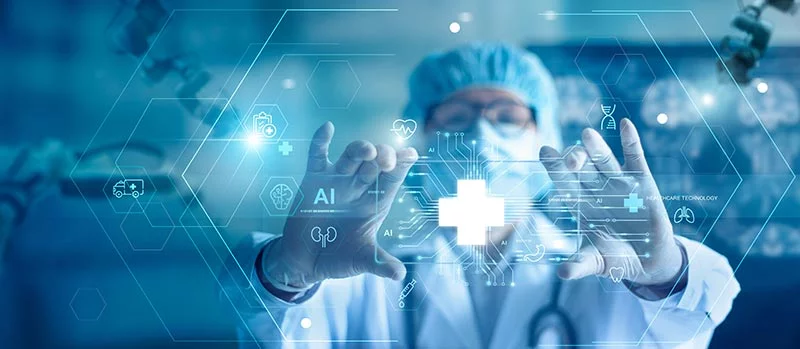
In a famous and eerily prophetic segment of the 1968 film “2001: A Space Odyssey,” two astronauts aboard a Jupiter-bound flight receive news from HAL-9000, the craft’s operating computer, that a key piece of equipment is about to fail and must be replaced. The astronauts respond accordingly, only to find that HAL was not only wrong, but also denies the error and won’t follow the crew members’ directives. A human-computer power struggle that ensues leads to an astronaut’s death and HAL’s demise.
The scene foretells recent dire warnings from some that artificial intelligence (AI) of the type portrayed in “2001” could endanger the human race. The critics of AI – broadly defined as the use of computer systems to handle functions normally performed by humans – worry that it could make large swaths of jobs obsolete, become a potent conveyor of misinformation and disinformation and, like HAL, wrest decision-making power from humans.
However, these questions cloud ongoing work to unlock creative and beneficial uses for AI. That search is underway in the medical field. It’s still comparatively early days for AI in health care, but data scientists, researchers, and clinicians are probing ways that AI can help health care providers in various ways, including:
- Diagnosing and predicting disease.
- Assisting with clinical decisions.
- Easing health care providers’ workloads.
- Monitoring patients.
- Improving communication with patients.
Fueling AI in health care: a center for AI innovation
Progress in all of these areas is being made on the University of Colorado Anschutz Medical Campus and at UCHealth. The Aurora campus is home to the Center for Health AI and the CU Department of Biomedical Informatics, both committed to harnessing the power of medical data in the service of driving innovation to improve patient care.
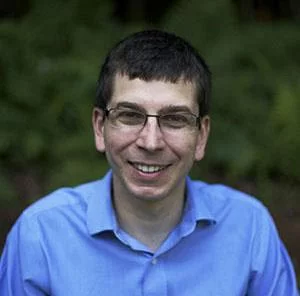
The reservoir of data available to be tapped is immense. For example, the Epic electronic health record (EHR) contains the detailed medical records of tens of thousands of UCHealth patients, while the Colorado Center for Personalized Medicine (CCPM) houses a deep biorepository of genetic information from a diverse patient population that is instrumental in investigating the roots of disease and developing targeted treatments for them. The Health Data Compass warehouse brings together data from CCPM, electronic health records from UCHealth University of Colorado Hospital and Children’s Hospital Colorado and other sources.
“Leadership at CU and at UCHealth are aligned in terms of understanding that AI is going to transform health care,” said Dr. Casey Greene, who chairs the Department of Biomedical Informatics and directs the Center for AI Health. “Being at the forefront of it is going to be important, and the question is how do we operationalize that general concept into practice?”
To that end, Greene said the Department of Biomedical Informatics provides an avenue for faculty with not only an expertise in computation and data analysis but also a desire to apply those skills toward improving patient care.
“When we recruit faculty, we want people who are leaders in their field and who are driven to see their impact in day-to-day changes in health care,” Greene said.
The investigations of providers and researchers on the Anschutz Medical Campus demonstrate both the burgeoning power of AI and the challenges it presents in the health care field.
A revolution in data collection and processing transforms health care
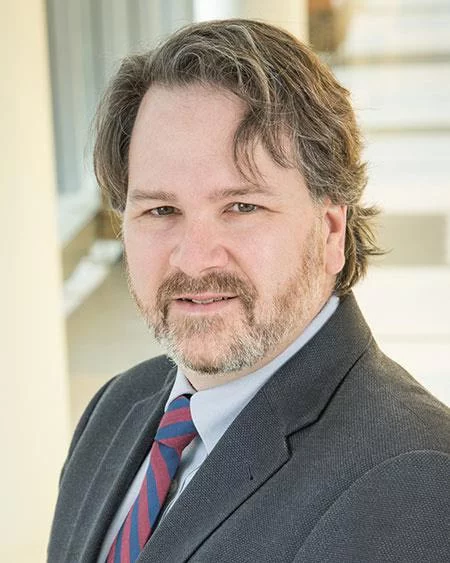
As the HAL episode of “2001” demonstrates, the concept of AI is not new. Its potential to spur changes in our lives soared with major advances in data-processing technology, said Dr. Toby Cornish, associate professor and vice chair for informatics in the CU Department of Pathology.
“We can now process large amounts of data in reasonable times,” Cornish said. Advances in parallel computer processing, the availability of affordable computer memory, and the development of graphics processing units that can efficiently handle large batches of images and other factors have enabled systems that can rapidly comb through deep stores of information in EHRs and other repositories.
For example, clinicians who have questions about, say, vaccination rates among particular patient populations, lab tests given to patients on high-risk medications or patients at risk for a particular disease can scour the EHR for the data in relatively short order and use it to help make decisions. As UCHealth Chief Medical Informatics Officer Dr. CT Lin noted, such tasks not long ago would have required a small army of people poring through paper charts.
“The EHR is a complementary tool that can detect helpful trends and pull out interesting bits of data,” Lin noted. “It’s an excellent assistant to the clinician.”
The adoption of AI in health care: Training the system to look for trouble
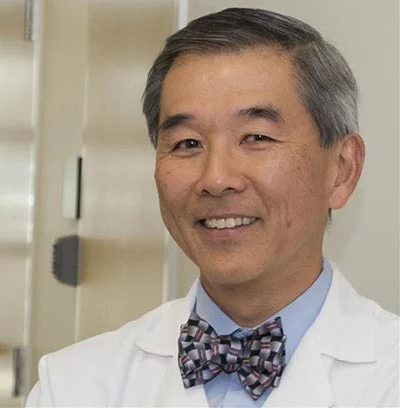
Powerful computing has enabled rapid advances in machine learning: the concept of setting up algorithms that train a system to look for patterns in the data it encounters. One important example: the UCHealth Virtual Health Center (VHC) remotely monitors patient vital signs, while AI assists in looking for patterns in heart and respiratory rates, fluid levels, blood pressure and other factors that could signal an impending spiral into sepsis – even before a busy bedside provider notices it.
The VHC evaluates the AI warning data, separates the false positives from the true signals of danger and notifies the bedside providers only when it is appropriate. Early detection allows the team to promptly start a sepsis treatment protocol that has kept some patients out of intensive care and saved lives.
“It’s a perfect match between AI and humans,” Lin said.
Cornish is also interested in using AI to detect patterns of disease that appear under the microscope. He collaborated with Dr. Fuyong Xing, assistant professor in the Department of Biostatistics and Informatics at the Colorado School of Public Health, to develop a deep-learning model – one that allows computers to learn a task using systems that mimic the processes of the human brain. The model can identify and count biomarkers of cell growth in neuroendocrine tumor cells automatically while ignoring intermixed normal cells. It’s a task that traditionally relies on a pathologist examining slides and manually counting the unhealthy cells. The time-intensive exercise leads to calculating a ratio that determines the grade of the tumor, a key element in developing a treatment plan.
Cornish and Xing’s work, published in a chapter of the book Artificial Intelligence in Medicine, is an example of what Cornish called “indirect prognostication”: AI assumes one part of the job he does himself as a pathologist in grading a tumor. A further step – still on the horizon, Cornish stressed – would be for AI to use tumor cell characteristics to directly predict what should be the follow-up care for patients or what their five-year outcomes will be.
AI to help medical professionals make informed decisions
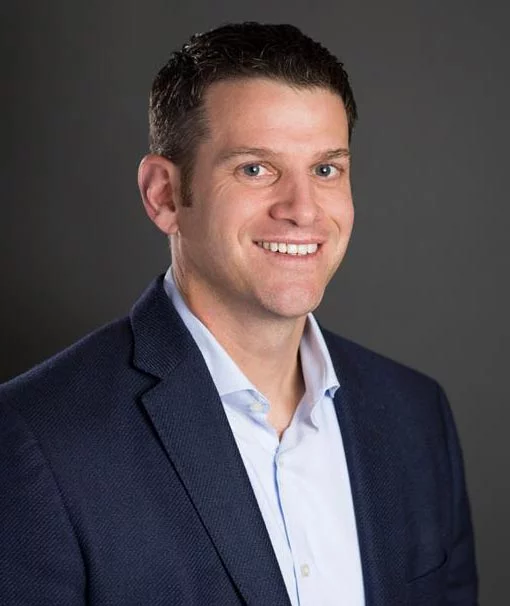
The use of AI for clinical decision support – such as developing a prognosis and treatment plan, as Cornish suggests – is another broad area of investigation. Dr. Michael Rosenberg, a cardiac electrophysiologist at CU, and his colleagues have examined deep-learning models that use electronic records from the Health Data Compass warehouse to predict those at greatest risk of developing a life-threatening heart rhythm disorder called QT prolongation after taking one of more than 100 medications. The information could be used to warn prescribing providers of the danger.
“The problem affects all specialties,” he said. “As more and more new drugs come out, we find this is something we have to manage. We could avoid giving the drug and after the fact realizing that the patient had toxicity.” Providers could also closely monitor patients who have no choice but to take certain anti-arrhythmic medications that increase the risk of QT prolongation, he added.
Rosenberg stressed that his decision support work involves a lot of trial and error that is still underway. But he said he’s excited by AI’s ability to help researchers and clinicians analyze massive amounts of data, as well as automate basic tasks, such as reading electrocardiograms to identify normal findings and help providers focus attention on possible problems.
“I’m optimistic we can do both to make health care better,” Rosenberg said.
AI satisfies the need for speed – and accuracy
Many medical problems require clinicians to apply exacting expertise in a time-constrained environment. A good example is identifying cerebral aneurysms, or bulges that strain vessels in the brain. Neuroradiologist Dr. Justin Honce helped to develop a system that uses AI to quickly review images for signs of abnormalities that indicate aneurysm risk. That could provide crucial help for both general radiologists and neuroradiology specialists, Honce said.
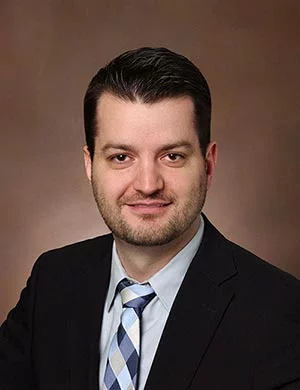
“Aneurysms can be hard to see because they can hide behind the branches of the vasculature,” Honce said. “For a general radiologist, AI can help define the aneurysm and ensure that it isn’t missed. AI can also help neuroradiologists find aneurysms faster, so we can read the studies and get the results more quickly to patients.”
Honce added that AI algorithms are also an emerging tool for detecting brain hemorrhages and vessels that have been blocked by oxygen-starving blood clots. Faster detection leads to quicker treatment to remove the blockage, restore blood flow to the brain and salvage tissue.
Overall, Honce said, radiologists face heavy workloads that require a balance between speedily reading cases while ensuring accuracy. “AI tips the balance in favor of both speed and accuracy,” he said.
Honce added that a future AI-related improvement will apply hemorrhage-detection software that is now used in the emergency department to outpatient exams. In that setting, a CT image of the brain flagged by AI with a possible hemorrhage will go to the top of the radiologist’s work list to accelerate diagnosis and treatment. The change is slated for the next upgrade of the hospital’s Picture Archiving and Communications (PACS) system, Honce said.
AI learns the language of health care
The PACS change is just one example of AI pointing to the future of health care. One key area: using large language models – those trained to synthesize and generate new information from data sets – to assist both providers and patients. They include:
- Training AI to “read” the dictated findings of a radiology study and summarize the details in an impression, which includes the diagnosis and possible treatment recommendations. Honce said the innovation could save radiologists an hour a day to read more studies.
- Generating draft automatic replies to incoming patient messages and questions for clinician review. It’s a tool that Epic is working on for its EHR, Lin said. “It’s like having a smart medical student,” he noted, adding that testing shows the tool generating useful replies for questions about straightforward cases, like an ankle sprain. Responses to more complicated issues, like CT scan results, are on the far horizon.
- Simplifying summaries of clinician findings in patient-friendly language. Honce said he is working with the Patient and Family-Centered Care Advisory Council at UCH on a project that uses ChatGPT – the most discussed of many AI large language models – to create reports that are easier for patients to understand. He thinks the work could “improve patients’ ability to ask questions” and give them “a better arsenal to ask questions.”
AI poses questions for the future of health care
The future of AI in health care is not entirely rosy, and Casey Greene, for one, urges “tamping down expectations” during this time of rapid change. For example, he notes, steps must be taken to ensure patient privacy is not breached, that the data the technology generates is used ethically and that it is regularly and rigorously assessed for quality and its usefulness for large populations of patients.
Cornish points out, for example, that despite “an explosion of literature” investigating the use of AI in gastrointestinal and pancreatic pathology, the approaches have not become best practice. The problem is that a model that performs well with its training data set may not duplicate the success on a new data set, he explained.
“We need a lot of evidence,” Cornish said. “Almost all machine learning methods must be validated against external data sets. Can the successes we demonstrate be generalized?”
Answering that question requires that physicians and other clinicians play a key role in developing the models that use AI, Cornish added. Their clinical knowledge is necessary to establish the “ground truth” for training models and validating AI performance, he said. Without that, the findings are meaningless or even dangerous.
“A computer scientist or an expert in machine learning is not going to be providing the subject matter expertise that is required,” Cornish said. “It’s really a partnership to build good models and to ensure that they are responsibly deployed.”
“For all of AI, it’s not really going to be useful until the clinician seeing patients figures out how to use it,” adds Michael Rosenberg. “The ability to bring data science to the hands of clinicians is really powerful and I think that’s where some of the innovations are going to come from – clinicians who find a problem and realize that the data is going to help them solve it.”