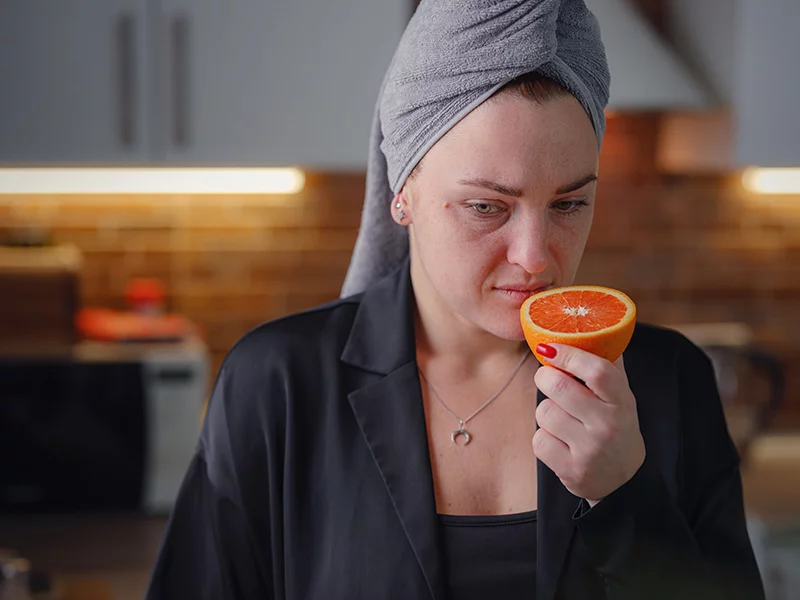
More than two years after the COVID-19 pandemic began, the disease has taken a staggering toll on human life. The number of deaths in the United States alone is approaching 1 million, while many more survived only after long hospitalizations and stints of rehabilitation. Just as troublingly, many others endured a COVID-19 infection only to find themselves struggling with symptoms weeks later. These long COVID patients, or long haulers, may encounter any of a bewildering array of ailments, including breathing problems, chest pain, chronic fatigue, brain fog, and many more.
The sheer variety and unpredictability of how the issues affect people presents a big challenge: how to define long COVID? What are the factors that point to an individual being a long hauler, or at least at risk of being one? If those elements could be identified, medical providers could point patients more efficiently to appropriate care for their symptoms, just as they would those at risk for diabetes, hypertension, and other chronic conditions.
A deep data dive into the roots of long COVID
A group of researchers, including many on the University of Colorado Anschutz Medical Campus, recently completed an initiative to meet that challenge. Their strategy: use a large electronic health record (EHR) to glean rich data about COVID-19 patients and identify those with characteristics that put them at risk for long COVID. The researchers took the deep data dive into the EHR repository with the aid of machine learning – that is, training programming systems to rapidly comb through mountains of data in search of clinical nuggets that illuminate the mysteries of long COVID.
The work produced a paper, “Who has long-COVID? A big data approach,” that was recently peer-reviewed and accepted for publication by Lancet Digital Health, said co-author Dr. Tell Bennett, head of the Informatics and Data Science section in the Department of Pediatrics at the University of Colorado School of Medicine. The paper was also the first produced by the National Institutes of Health-funded RECOVER study, which is recruiting patients nationwide to study long COVID, Bennett said.
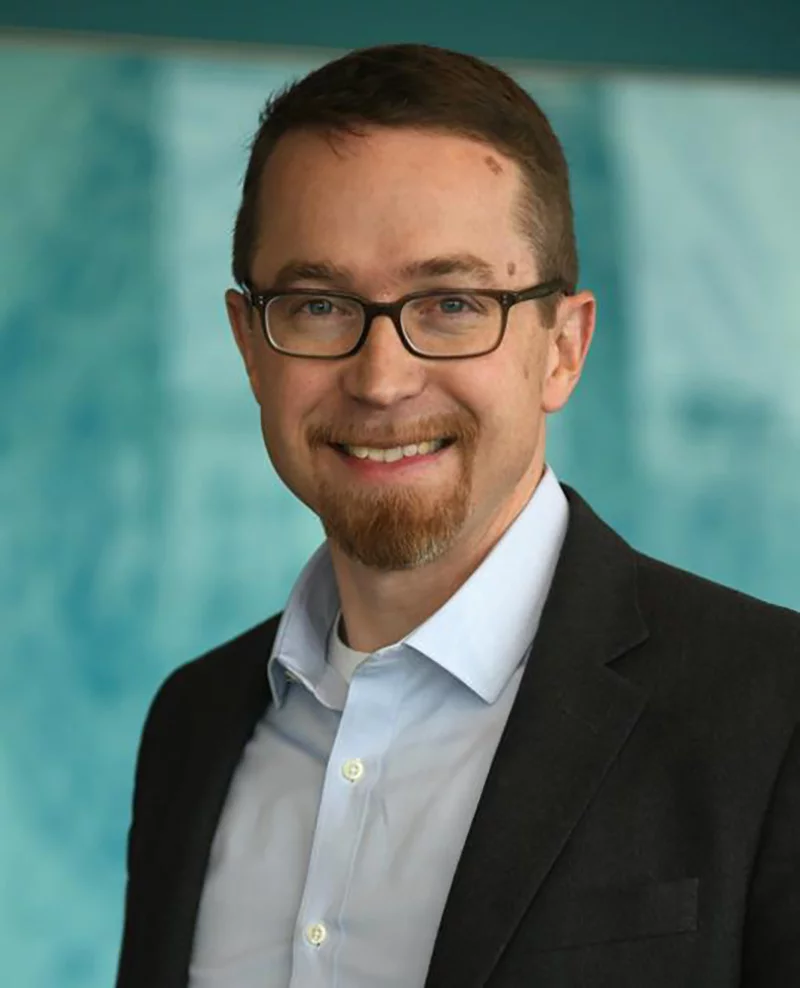
Many months after patients began reporting long-term struggles with post-COVID symptoms, providers have learned more about it through treatment and observation. But tapping a large repository of medical records promises to sharpen the clouded picture of a condition with various physical and psychological symptoms that often overlap.
“The EHR is especially good for investigating long COVID because we are trying to define what is essentially a new entity,” said Dr. Sarah Jolley, assistant professor of Pulmonary Sciences & Critical Care Medicine at the University of Colorado School of Medicine and medical director of the Post-COVID Clinic at UCHealth University of Colorado Hospital. Jolley is a co-author of the study.
Defining the tell-tale signs of long COVID
The big data plunge yielded the first phenotype, or set of identifiable characteristics, for long COVID, Bennett said. Those characteristics include increased health care utilization, age, shortness of breath, difficulty breathing and particular diagnoses and medications patients received for the first time at least six weeks after their acute illnesses.
The phenotype, which researchers will continue to hone with additional data, opens important doors in the understanding of long COVID, Bennett said. First, it can help the RECOVER trial reach its goal of recruiting some 17,000 patients across the United States to study not only long COVID patients but also those who recovered without long COVID and healthy controls. University of Colorado Anschutz Medical Campus is part of a consortium of universities and hospitals involved in that initiative.
Second, having a phenotype helps researchers and clinicians to develop hypotheses about not only the risks for long COVID, but also possible treatments and therapies to test in future trials, Bennett said.
Machine learning scales mountains of data in search of a long COVID definition
The study’s strategy for using machine learning vastly increases the chances of meeting the challenges of studying long COVID, whose diversity of symptoms has made it difficult to form a workable definition, Bennett said. For example, the World Health Organization (WHO) compiled a list of 12 “domains” that formed its definition of “post-COVID condition.”
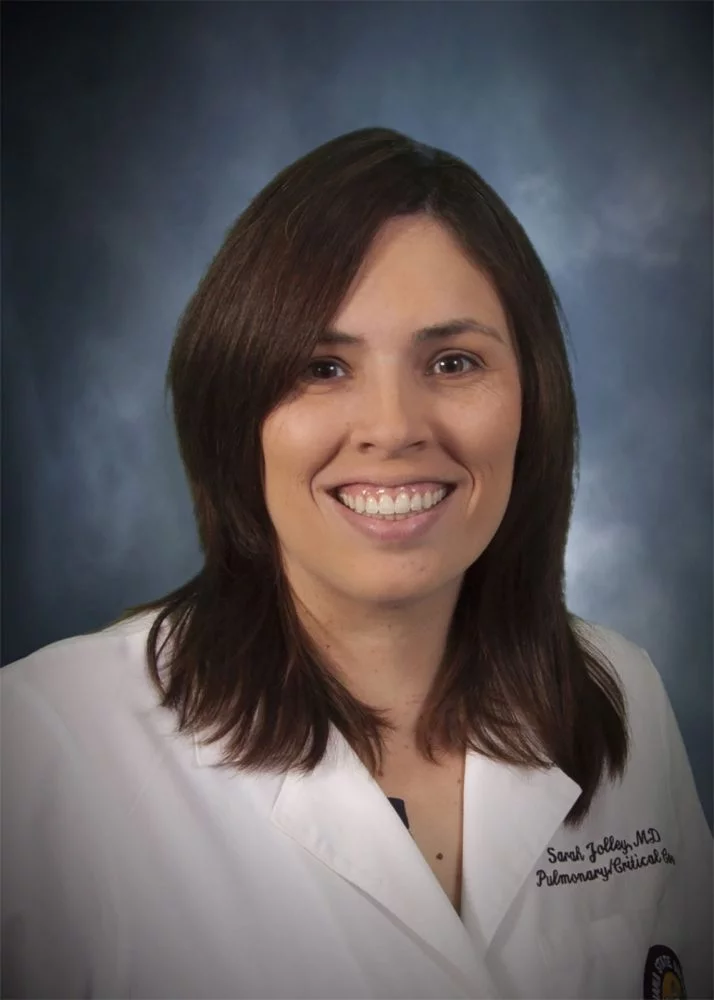
The WHO definition encompasses lab confirmation; minimum times for onset, duration, clustering and number of symptoms; complications; and its effects on everyday functioning. It also aims to apply the definition separately to children and other populations. The breadth of the definition makes it very difficult to scale, particularly when there are tens of millions of people around the world who have had or are getting COVID-19, Bennett said.
“You need to find a way to winnow down to the people who would most benefit from or be most willing to participate in a clinical trial,” Bennett said. That’s always an arduous task. But machine learning makes it easier to dig through layers of detailed electronic medical records in search of those patients, he added.
Technology lends research a hand
The development of the big data study is in itself a story of how to turn technology against a stubborn disease foe. The research team used the EHR of the National COVID Cohort Collaboration (N3C) to look at information about health care utilization, demographics, diagnoses and medication use from nearly 100,000 adult patients with COVID-19.
The researchers used data from that group and nearly 600 patients who received care from long COVID clinics at three sites, including the UCHealth Post-COVID Clinic, to train their machine-learning systems to identify patients in the N3C database at risk for long COVID. The system probed all COVID-19 patients, those who had been hospitalized after infection, and those who had not. The characteristics of the patients identified by machine learning as potential long COVID patients correlated closely with the patients who were treated at long COVID clinics.
In sifting through a large number of potential characteristics of long COVID, the system returned those that were “the most powerful in terms of predictive value,” Bennett said. These formed the basis of the new phenotype. The work identified some 100,000 patients in the N3C database who were potential long COVID patients.
Still in search of a long COVID “gold standard,” but getting closer
A firm definition of long COVID – a gold standard – still awaits, the researchers said. But in its absence, the characteristics of patients who received care at a long COVID clinic served as a “silver standard,” or “a valuable proxy for long COVID until a true gold standard is available,” as they put it in their study findings.
For Jolley, the EHR work also helped to validate what she sees in treating patients in the clinic.
“What we saw in the big data cohorts mirrored what we were seeing clinically in terms of risk factors and symptom patterns,” she said.
Of course, the ultimate goal of the technical work that produced the study is to help as many patients as possible. Jolley noted that solidifying a definition of long COVID will make it easier to craft “clinical pathways,” or established, evidence-based treatments for specific symptoms, broadly available to medical providers in different areas of the country.
“Using the EHR to inform those pathways will increase access to more standardized post-COVID care, particularly in rural and underserved areas where patients may not have access to a specialized long COVID clinic,” Jolley said.
More information to clear the mysteries of long COVID
The work could also spur development of “clinical decision support” tools in EHRs to aid providers treating patients with long COVID symptoms, Bennett said. The EHR would produce an alert if a patient’s record shows risk factors for long COVID and help the provider connect the patient to additional resources, testing and treatments, he said.
Jolley said she also hopes the big data approach will help to clear some of the mystery, fear and misunderstandings that have shrouded long COVID. The difficulty of defining it precisely has sometimes made providers and others skeptical of those suffering through its symptoms, chronic fatigue and brain fog being two troublesome examples.
“For some providers who don’t see long COVID patients as frequently as we do, some of the symptoms are not as obvious or evident,” she said. A more precise definition of the condition, she added, will be “helpful to increase the awareness of its spectrum and will let providers know if patients are presenting with these symptoms, they should be believed.”
The same might apply to employers who are puzzled when an employee takes longer than expected to recover after a COVID-19 bout, Jolley added.
Definition of long COVID is likely to evolve
Moving forward, Bennett said “a vision for the future” is to sharpen the long COVID phenotype and generate simplified versions of machine learning programming that might run on a tablet or web server. That would help providers who don’t have access to a sophisticated EHR identify patients at risk for long COVID. With that they might evaluate patients with specific symptoms – pulmonary function tests for those with respiratory problems, for example – or refer them to specialty care, Bennett said.
“If our estimates turn out to be accurate, there could be large numbers of long COVID patients. We need to empower providers with multiple levels of information to take care of them,” Bennett said.
Finally, the study concludes that pinning down a long COVID definition will likely continue to be challenging.
“It is plausible that long-COVID will not ultimately have a single definition, and may be better described as a set of related conditions with their own symptoms, trajectories, and treatments,” the authors wrote. Thus, long COVID patients may “cluster” in “sub-phenotypes” with characteristics that reflect their heart, nervous system, pulmonary, mental health and other issues.
The big data study did well to “identify the big picture” of long COVID, Jolley said. But she acknowledged that the broad view is made up of many complex details.
“The consensus is that there probably is not one unifying diagnosis, but subgroups, with some overlap,” she said. “With such a heterogeneous condition, getting to one single definition has been harder than expected.”