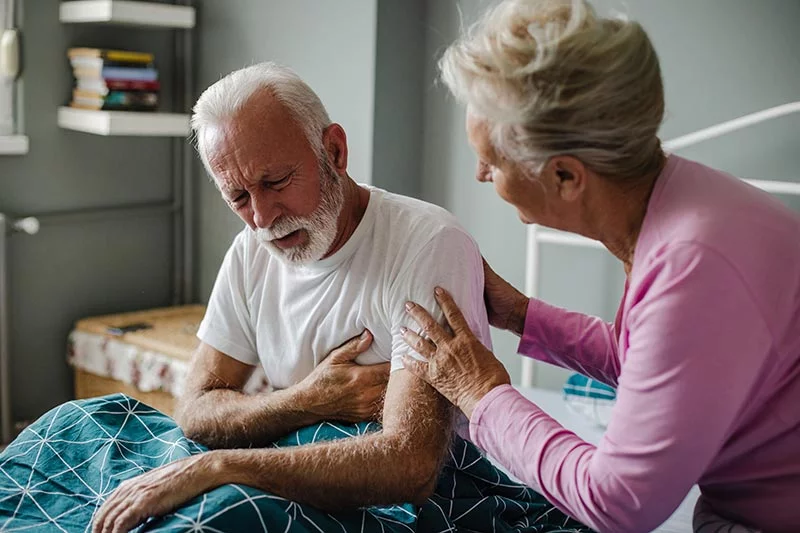
Some mashups of old and new don’t quite work: horse carriages and reusable rocket boosters, say. But combine a 19th-century medical advance with 21st-century computing technologies, and one now has the ability to identify patients at high risk of atrial fibrillation up to a year in advance. That breakthrough, clinicians hope, will help prevent thousands of strokes that hard-to-diagnose Afib causes each year. It also could spot structural heart diseases earlier, improving outcomes through more timely treatment.
The old technology in question is the electrocardiogram (ECG or EKG), a heart-voltage detector invented in 1895 that’s been a low-cost, mainstay cardiac diagnostic pretty much ever since. The modern computing technology at play involves machine-learning algorithms developed by Chicago-based Tempus and Pennsylvania-based Geisinger Health. Those algorithms feed into a convolutional neural network that interprets the waveform – the shape of an ECG’s many spikes, dips and subtle undulations – in ways no human ever could.
Tempus’s artificial intelligence (AI) works on the same basic architecture as what YouTube uses to scan image data to identify cat videos, or self-driving cars use to identify objects in the road, explains Noah Zimmerman, Tempus’s vice president for translational science.
“An EKG is measuring voltage, right? We look at those voltages and treat them almost like image data,” he said
While studies in such journals as Circulation and Nature Medicine have shown this old-plus-new approach to work surprisingly well – to the point that the U.S. Food and Drug Administration is fast-tracking the technology – the AI tool needs more testing. Further, once that training has Tempus’s ECG Analysis Platform ready for prime time, the new diagnostic must be incorporated into health care processes so doctors can make the most of it for their patients. In pursuit of those ends, Tempus is partnering with the UCHealth CARE Innovation Center.
Partnering to predict atrial fibrillation and structural heart diseases
That partnership is proceeding in three phases, says Emily Hearst, UCHealth’s Tempus projected manager. The first is looking retrospectively at the ECGs of 5,000 UCHealth patients and seeing if Tempus’s ECG Analysis Platform can repeat the sort of results it has delivered before. That Circulation study involved feeding the ECG Analysis Platform 12-lead digital ECG traces from 430,000 patients collected from 1984 to 2019. Using historical data allowed Tempus and Pennsylvania-based Geisinger Health to see how the AI system’s Afib predictions tracked with future Afib-related strokes. The system spotted nearly two-thirds of patients with no documented history of Afib (Afib being episodic and often without symptoms, it can go undetected for years) but who later had an Afib-related stroke.
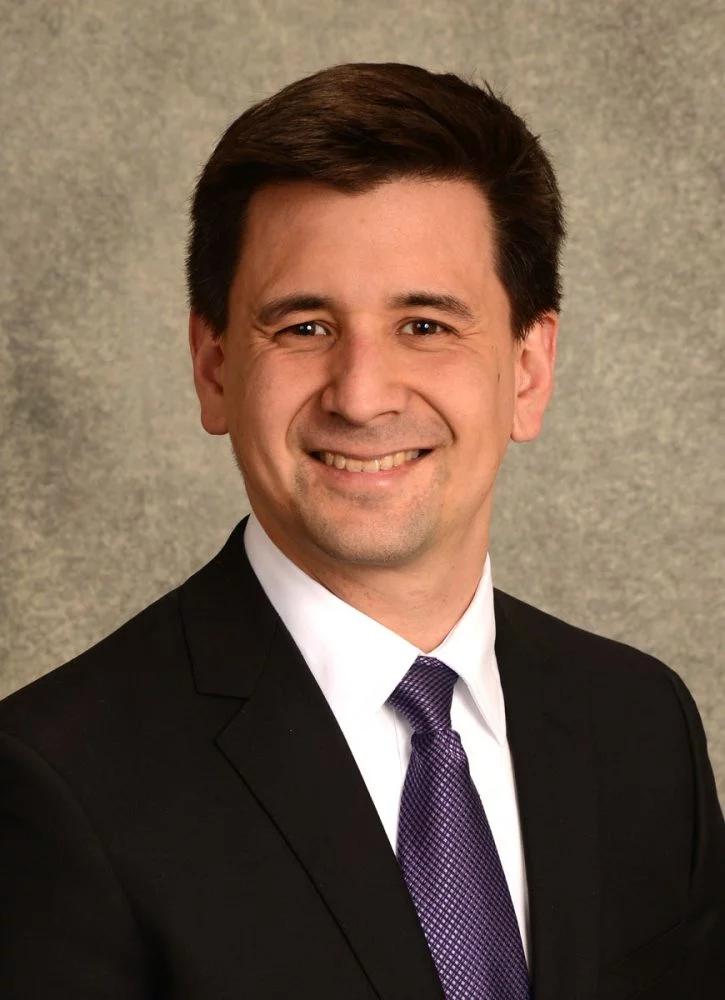
Why would examining a fraction of as many patient ECGs as Tempus already did help prove out – much less improve – the platform? Dr. David Kao, the University of Colorado School of Medicine and UCHealth cardiologist who is working closely with Tempus, says it’s about diversity. People are people, but the population makeups of Pennsylvania and Colorado differ. An AI-based system’s intelligence must reflect that.
“Overfitting is a huge problem in machine learning, which means it can perform very well in your initial, however-large dataset, but then it doesn’t work anywhere else,” Kao said.
What’s called an external validation set, one using a different patient population than the initial training set, can both refine the model and lend its creators as well as regulators more confidence in the prospects of its real-world performance, Kao says. In this case, the results, if they’re favorable, will strengthen Tempus’s FDA submission for full approval, Hearst adds.
Beyond predicting Afib
The second phase of the Tempus-UCHealth partnership is boosting the number of previously recorded patient ECGs fed into the Tempus system to 45,000. The goal will be to validate results from a recent study that showed the model to be capable of using those same ECG traces to predict structural heart diseases – a group of conditions that adversely affect the valves, walls, chambers, or muscles of the heart such as aortic stenosis and hypertrophic cardiomyopathy.
The third phase of the partnership will also look at structural heart disease, Hearst says, but prospectively – that is, feeding the Tempus platform ECG readings of current patients and seeing how well it predicts structural heart disease going forward. Should the results of one these studies pan out, UCHealth and Tempus will work on how to integrate the AI-based results into UCHealth’s – and, by extension, that of many other health systems – electronic health record, Hearst adds.
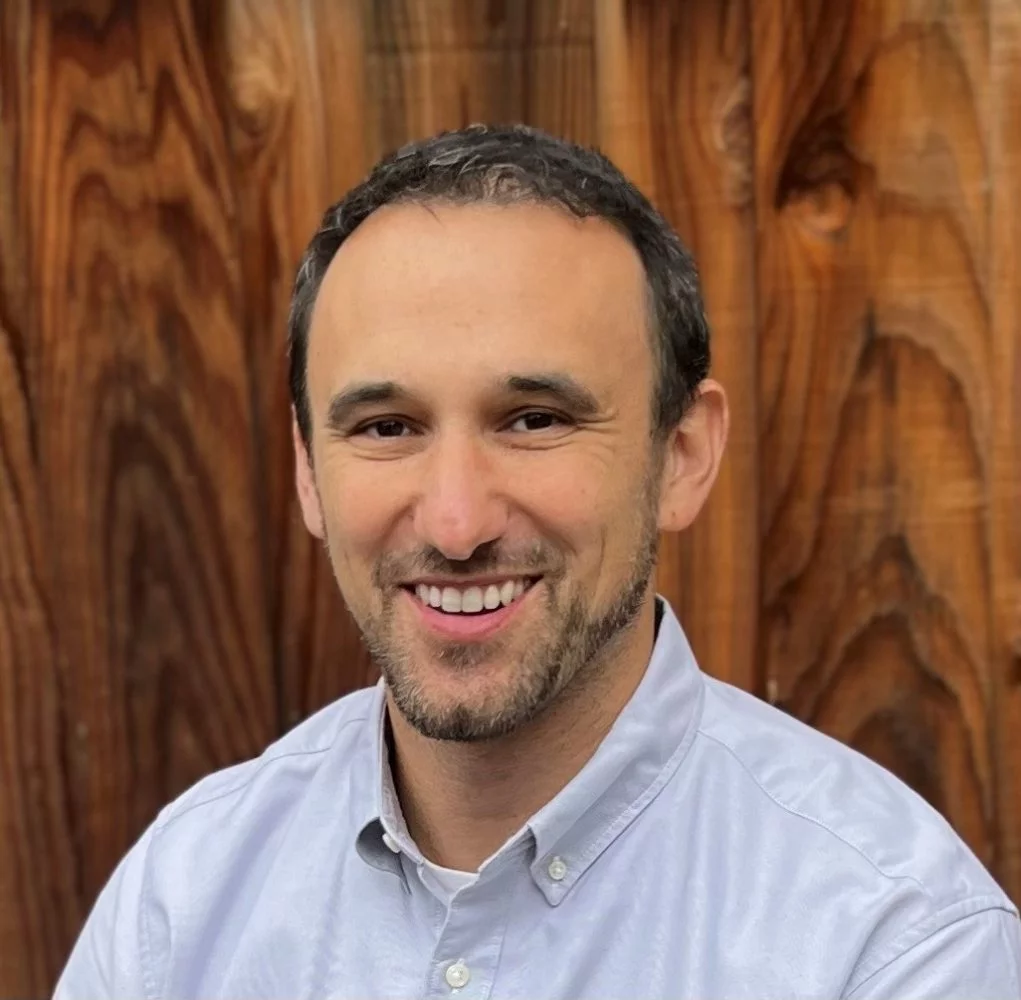
Success could save countless lives and could have a particular impact on underserved communities in the United States and entire countries abroad. Kao, who has done medical trips to Zimbabwe, says that country has, in the past, had one or two 3D-ultrasound echocardiogram machines of the sort that cardiologists use to diagnose serious heart problems. It takes specialized training to interpret echocardiograms, and the machines themselves cost tens of thousands of dollars. Combining the outputs of an electrocardiogram machine that costs $500 to $2,000 with AI that can spot patients at high risk for Afib or structural heart diseases could identify those who would gain from preventative treatment. In places where cardiac specialists and higher-end diagnostics are available, ECG-based AI results filter patients such as those most likely to have problems see specialists first, Kao says.
Kao adds that he considers partnerships such as UCHealth’s and Tempus’s as an exemplary innovation model, one which combines the rigor and clinical experience of academic medicine with the expertise and commercial motivation of industry.
“I don’t know that one or the other can do it on their own,” he said. “You need the strengths of both. It’s hard to find partners that line up, but when you do, it’s like lightning in a bottle. You’ve got to hold onto it.”
The old and the new may not always harmonize, but Tempus, with a big assist from UCHealth, appears to be playing a tune that could help patients until AI is old hat, too.